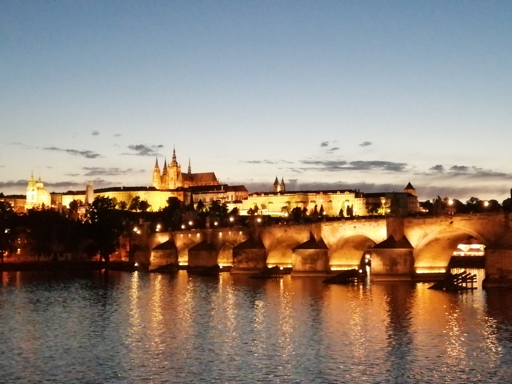
Before my memory fails me – I didn’t take notes – I wanted to write down whatever I remember about the IACAP conference that took place in Prague in the beginning of July.
There was a certain old vs. new guard feeling pervading the whole conference which played out mainly between traditional philosophy of computation and information and the new approaches to philosophy of AI/ML.
I certainly wasn’t the only one who had this impression, several members of the old guard bemoaned the spread of AI ethics – apparently to the detriment of philosophy of information. I myself am unsure if this is just an outflow of the current AI summer or if these problems are here to stay.
In any case the juxtaposition of AI ethics and philosophy of computing was by no means accidentally as evidenced by IACAPs picks for this year’s senior (Covey) and junior (Simon) awards: Oron Shagrir and Kathy Creel. And great picks they were!
Oron delivered his price speech on how neural nets changed the concept of physical computation – ontology showing itself to be a true old guard’s favourite. If I remember correctly (should have taken notes) he argued that recurrent neural networks – a method that has not gained much traction yet in the ongoing deep learning hype and should not be confused with deep neural nets – vindicate the semantic view of computation. Of course Oron has argued for the semantic view before, but this seems to be a different line of argument yet.
Kathy gave her talk on homogenization in ML methods (based on this paper), representing the new guard with their focus on ethics of AI.
She connected algorithmic monocultures, that is the usage of closely related algorithms by e.g. different companies for applicant selection, to dangers of homogenization. The idea is this: if you get misclassified as a non-worthy applicant by an applicant selection ML-model M_1, you are also likely to be misclassified by the closely related model M_2 and so forth.
M_1, M_2,… will likely have different accuracies, so it is not clear if the misclassification is to blame on the lack of accuracy of the model in question or some other property of the applicant the models latch on. To factor this out Kathy and collaborators proposed a homogenization measure which is basically the ratio between the probability of all the models failing for the applicant in question and the probability that randomly sampled outputs for each of the models fail.
She suggested that companies interested in combating the problem of homogenization could wiggle the decision boundary in borderline cases randomly thereby counteracting the effect of algorithmic monocultures.
This is of course not only possible for the companies themselves – who might not have any interest in doing that – but also for the candidates. They could just slightly randomize their application data to the same effect. I think it is important to realize that the subjects of algorithmic decision making can be empowered to counteract certain negative effects themselves without relying on the good will of whoever employs the algorithm.
Concerning the homogenization measure I have my usual worries about a tacit iid assumption that goes into estimating the probability of model error. To estimate that probability one needs to assume an error distribution for each of the models, exactly the ground truth that is not available in most high stakes contexts.
There were lot’s of interesting talks of which I will mention only a few in passing, because they somehow stayed in my mind.
Luis Lopez presented the idea that ML methods should be viewed as approximation methods (something I could not agree more on) and that this would resolve many (artificial) problems in the epistemology of ML. I hope he develops this idea further.
Ashley Woodward gave a very learned and convincing talk about continental approaches to philosophy of information, where he argued that there was a philosophy of information developed in parallel to the analytic tradition and presented some specific problems continental approaches share. In this context it might be interesting to note that Floridi himself renounced analytic philosophy recently (see his reply to Gabriel).
There was a very interesting symposium on Turing and Ashby on Computation, Mechanisms and Intelligence by Hajo Greif, Adam Kubiak, Paula Quinon and Paweł Stacewicz. I knew that Ashby played an important role in early cybernetics, but I learned that he had quite different views on the nature of computation than what has become the ruling orthodoxy.
I hope Ramón will write something about his own talk on AI as an epistemic technology. He promised that viewing AI as such will solve several long standing puzzles about AI – for example which nature trust for AI has. The talk sparked a very lively debate on the definition of epistemic technology.